Four steps to building a data driven marketing strategy: part 3
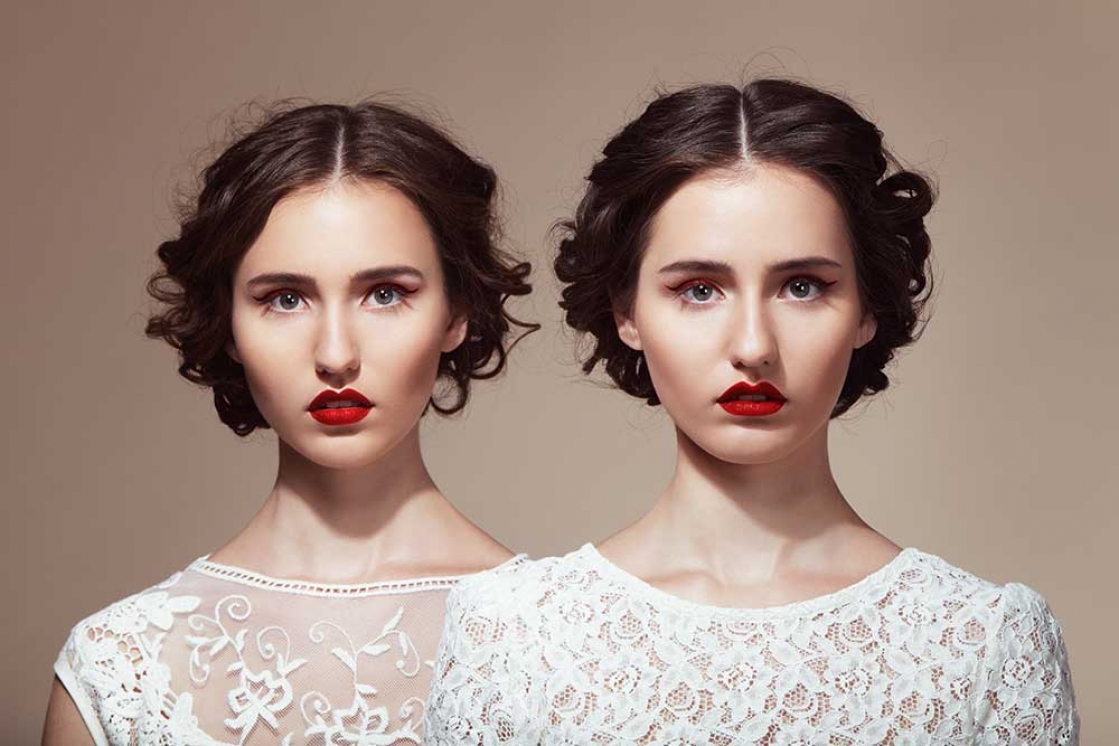
Part three in our four-part blog series looks at how to combine your own customer data with that of a third party to better tailor your marketing strategies.
PART THREE
Look-a-likes: how to segment your customers
In part two we looked at how third-party data can provide better insights into your customers by filling any gaps and strengthening data. Assuming you’re now armed with first and third-party data, it’s likely that you’ll be sitting on some rich pickings.
But how do you best combine the data and use it to drive decisions?
The answer lies in segmentation; grouping look-a-like customers by key criteria.
Segmenting by gender is fundamental to marketing strategy. As well as telling you the obvious needs of male vs female, it also ensures that you’re offering relevant products or services to customers. You’re unlikely to get engagement from men if you’re sending them offers for female hygiene products. Recognise that the needs and wants of men and women differ on many levels but ensure that your data leads you rather than assumption.
Segmenting by age means you can target customers with age appropriate offers. Whilst some products are relevant across all ages, many are not. Insurance is a classic example as consumers often need different types of insurance at different ages. Renters insurance, for example, will often be targeted at a younger age group, whilst mortgage protection insurance will likely be guided by data that shows the average age of the purchase of a first home.
Segmenting by income means grouping customers by what they earn. Defining and naming income brackets will ensure you don’t end up with too many small segments. Income is one of the most important factors to consider as it will identify whether what you’re marketing is a need, want or luxury. This is because the buying power in each bracket varies.
Segmenting customers by where they live is a key tool in data driven marketing strategies. Where someone lives will affect their buying decisions. For example, customers living in a very hot climate will have little or no need for heating whilst the opposite is true of those living in a very cold climate. This is a multi-pronged segmentation strategy as you will also need to incorporate key information about each area, rather than just a name.
Segmenting via occupation: Occupation is a good indicator of income and will influence the purchase decision of a customer. Grouping customers via occupation will identify similarities and highlight opportunities for personalised marketing. For example, what might be a luxury for those in certain occupations may be a mainstay for others. Depending upon your business, segmentation via occupation can also help you sell occupation-specific products.
Segmenting via usage is particularly valuable to utility companies. If you’re an internet services provider, you can group your customers by the amount of data they use. For those that sit in the heavy user group, you may be able to offer another more relevant data plan that meets their needs.
Segmenting by lifestyle requires a deeper dive into your data. Grouping via marital status, interests, hobbies, values and more is just one way in which an external data provider can help. If you don’t have this information from your customers, data from social media, online behaviour and purchases will support more accurate segmentation.
Once you’ve got the basic segmentation strategy sorted, you’ll be able to further analyse subsets for more granular detail. Getting an external data provider on board will allow you to see your segments in more detail.
For example, you may have identified a high-income group that seems perfect for a premium credit offer. Whist it might be tempting to market to the entire segment, it pays to cross reference with other external insights. Applying credit history to this group may identify outliers or those who sit outside that group. What will often differentiate them from the rest of the segment can be as distinct as a payment default.
The final part in this blog series will look at the implementation of data driven marketing, examples and how to measure results.
Meanwhile, download our white paper on customer segmentation for more information.